Field report: AI & ChatGPT in qualitative research
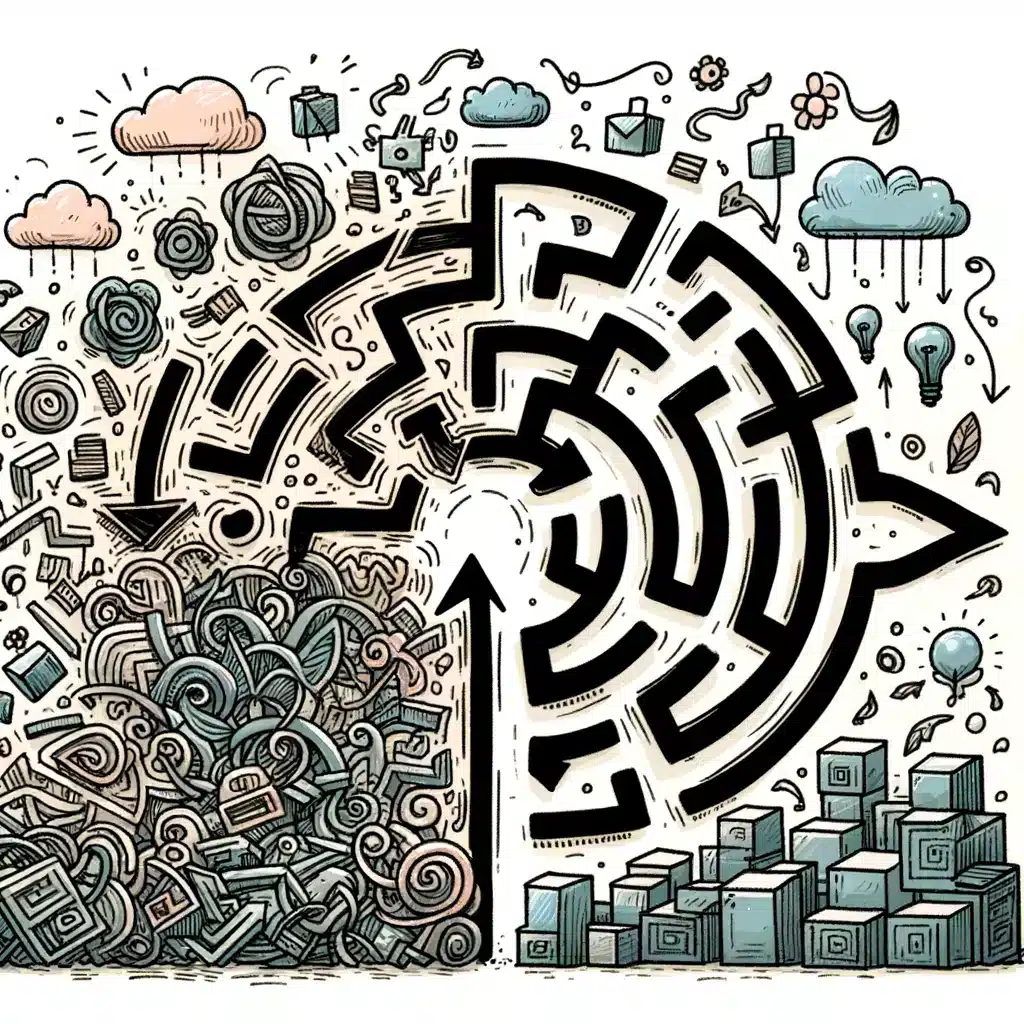
AI – a new tool in qualitative research
Qualitative research is challenging. Researchers must develop a suitable research question and select and collect the necessary data. They then need a strategy to analyze the data, understand this strategy and implement it correctly. Finally, they have to prepare, interpret and, if necessary, defend the results.
Researchers have been receiving support in each of these areas for decades. This support comes from research literature and methodological instructions, from exchanges in research workshops, supervision by experts and peer review. Tools such as pen and paper or QDA software also help. This support ensures that the work processes in qualitative research are clearly regulated, transparent and often easier to carry out. These tools are now potentially being joined by artificial intelligence (AI). In this article, we describe how we experience the current role of AI and what problems it can solve in the context of qualitative research. This is a snapshot from June 2024.
AI solves a problem through automatic transcription
For 20 years, our core contribution to qualitative research has been to support the transcription phase. Both through methodological instructions (e.g.: https://www.audiotranskription.de/regeln/), as well as through the provision of transcription software and USB footswitches. The f4 programs have been available since 2005 with over 1 million downloads. Together with our transcription rules, they are an integral part of qualitative research and methodology at German-speaking universities.
We added AI to our range in 2019 with f4x automatic speech recognition. Especially since 2022, we have seen from the usage figures that the use of AI is fundamentally influencing and changing established usage habits of qualitative research at universities. There are around 100,000 f4x users, mainly from the university sector, who have automated many hundreds of thousands of hours of material (as of 05/2024). At the same time, the acceptance and use of manual transcription is decreasing. This is understandable, as automatic transcription saves around 50% of working time compared to manual typing. This frees up many working hours at universities, allows them to be used for other purposes or makes it possible to tackle certain projects in the first place.
The way researchers transcribe their interview data has changed substantially in the last 2 years thanks to AI. Why? While other AIs such as PI.ai and ChatGPT are very broadly designed to chat about a wide variety of text content and can therefore take on a potentially wide range of tasks, transcription AI is very specifically designed to “only” convert audio/video into text. Although there are also many interpretative and analytical processes involved in transcription (we have described this in more detail in the article“Why transcripts are never ‘correct’“), it is mostly or initially about the representation of the semantic content. And this can be clearly checked and evaluated. AI errors can be identified and corrected by proofreading. By listening to the recording and proofreading the transcript at the same time, you can identify the places where a word is missing or incorrectly placed. Assignments of incorrectly recognized speakers can also be identified and adjusted. And passages where the AI had a blackout and nothing was recognized or fabulated can be corrected manually by listening and typing. A transcript generated by speech recognition and then carefully corrected provides a solid basis for qualitative data analysis with a reduced workload.
The usefulness of using AI for speech recognition is therefore clearly visible and given in many contexts. AI solves an existing problem satisfactorily: AI facilitates the preparation of scientific transcripts because it offers plausible and verifiable results of work that could previously only be done manually, which takes less time overall to arrive at equally “good” data material.
What problems does AI solve in qualitative analysis?
What could be more obvious than the idea that AI could also simplify and accelerate other parts of qualitative research, such as the development of guidelines, data collection and the extremely demanding process of data analysis and presentation of results? What problems could AI solve here? Instead, you can take a look at the technical solutions that are already available as AI functions for qualitative researchers. In tools such as chatGPT, MAXQDA AIAssist, Atlas.ti, Ludra and others, we see offers in three areas:
- AI as a text generator. AI can generate text summaries and image descriptions. Other descriptive work, such as a paraphrasing or a formulating interpretation, can also be generated by AI. Suggestions for guideline questions and information on access to the field and the respondents can also be generated.
- AI as a coding machine. AI can make suggestions for topics and outline headings based on a given text selection. They can identify passages from given topics and try to match them.
- AI as a sparing partner. Chat AI can also act as a conversation partner to talk about the text content/images provided and ask questions about the material. We write about this here.
We have spent the last few weeks experimenting with chatGPT-4o, for example, and carrying out various tests. We personally really enjoyed playing with AI and discussing our own findings with AI. The results are often astonishing at first glance. This all takes place on a playful, experimental level. At first glance, many things seem immediately presentable, sometimes even impressively eloquent, and the speed with which this happens is impressive.
AI does NOT solve a problem through automatic analysis
Is it possible to simply upload material and get a reasonably meaningful analysis by asking ChatGPT a few questions? We tested this with existing material. We spent a long time reformulating and clarifying prompts, having known material evaluated and comparing it with the existing analyses we had prepared ourselves. For this purpose, we had ChatGPT create summaries and topic identifications.
The good illusion of the plausible result is the problem!
We have always experienced failures and errors with almost all summaries and topic identifications of different AIs (including the new GPT 4o):
- Text passages from the material were ignored
- Quotations were invented or incorrectly referenced
- The results were only produced for parts of the material or were incomplete in themselves
- Misinterpretations of our requests and hangers-on
- Am I just too stupid to formulate the prompt correctly? Come on, I’ll try it again (a few hours, days …)
These experiments were always accompanied by euphoria and hope. The impression “oh we’re sooo close…” was always replaced by the realization that something was still missing, an artefact had crept in or the material hadn’t been fully considered. Sometimes the amount of material to be considered was simply too much for the AI – without us receiving any feedback. The results were always presented as if everything was in order. And this is already the case with a typical amount of data sets of qualitative studies (e.g. 200 short questionnaires with open answers or 10 short interviews of 2-4 A4 pages).
The frequently discussed prompt engineering, i.e. the design of “good” requests to the AI, is often cited as an important tool for successful results. This is correct in principle, because imprecise queries lead to imprecise results. Here, too, the “principle of hope” has led to a long period of experimentation. But even suitable tricks, such as prefabricated models in GPT or the use of “mega-prompts” (generating prompts with the help of AI) and intensive refinement work have delivered incorrect or incomplete results. A bit like a needle in a haystack that you hope to find without ever knowing: If it’s enough, it’s better. The verification of the results was only possible by meticulous self-coding and recounting of quotations and checking every single source reference. This often enabled us to see that the AI results were incorrect and that something crucial had been forgotten.
And this is how everyone who wants to use AI for evaluation will currently feel. You are confronted with questions about how prompts are formulated appropriately, how the results of the AI are evaluated or whether an automatically generated assignment of text passages to a topic is exhaustive. We consider the verification work to be many times more time-consuming than direct manual analysis. The evaluation and verification of an AI-generated analysis requires analytical work, skills, knowledge, time, patience and is in this sense also additional analytical work. The supposed time advantage therefore does not exist for us in the context of scientific projects of qualitative research. For other requirements in other application contexts, this may be assessed differently (e.g. “I’m satisfied with a pi mal thumb”). Our conclusion: In a real research project, AI-based evaluation and massive time savings in the analysis process do not (yet) work “just like that”.
AI as an experimental tool for experienced researchers
AI is and remains totally exciting and we look forward to seeing how it is experimented with in research projects over time. However, these are – without any disrespectful undertone – experimental functions. These are functions that give experienced people a field of experimentation to explore the possibilities of AI and, if necessary, to report on them. People with experience in qualitative research can assess the results. They have opportunities for comparison, empirical knowledge and certain criteria with which they can evaluate the results and their “usefulness” in the respective field of research. Those who already have previous experience, who know their material and perspectives and are interested in experimenting will find exciting tools in the AI tools that are worth pursuing further. AI is used successfully and across the board when it can solve an existing problem satisfactorily. And it does this above all when the partial results of AI-supported work phases have a high degree of plausibility, are accurate and easy to check. This is currently not the case.
Open question data protection
We have so far left out the topic of data protection, although there are already fundamental objections to the use of AI here too. At least when it comes to dealing with interviews, we have a clear legal basis in Europe: the General Data Protection Regulation. This regulates how we may handle the data and what is not permitted. One aspect of this is that, unless there is explicit written permission for this, the interview data may not leave the scope of the law. If someone wants to use OpenAi Chat-GPT Tool or MAXQDA AIAssist, for example, data is often transferred outside the EU. This is generally prohibited for interview data. This must be examined very critically – each university has developed coordinated positions on this together with the respective data protection officer, which should be asked for or requested. Some universities even prohibit the use of these tools. You can find a detailed description of the issue at: https://sozmethode.hypotheses.org/2365
AI helps as a savings partner
To date, we have achieved the best and least data protection-critical support in the research process by using ChatGPT as a discussion partner. We used ChatGPT-4 to discuss questions or ideas about our material without revealing the actual research data. No specific names, places or other specific personal references were transmitted. In principle, we used ChatGPT as a sparring partner whom we asked for advice or with whom we discussed our ideas, similar to an interpretation workshop. This method has proven to be valuable for formulating and concretizing our own ideas and insights and for obtaining new suggestions for theoretical references.
UPDATE 19.06. Our impression is that Google’s “Gemini” is much better suited than ChatGPT. The answers seem less superficial and make more exciting references.
Concrete procedure
The first analysis step was carried out completely without AI. For this test, we chose a project on the topic of “Living in shared flats”, which we were already somewhat familiar with. We had read the material in its entirety and made memos on the individual paragraphs in which we questioned the statements (“What is happening in this sentence?” and other “W questions”). We looked for suitable overarching themes and assigned initial text passages to them. The coded original text with memos was opened in f4analyse.
Now GPT was added. We opened a separate browser window with ChatGPT-4. A central theme that we had previously identified manually in our material was “transition” (from “living at home” to “living independently”). In order to find out in which other research contexts research is being conducted on “transitions”, we used ChatGPT to gather information. It was able to provide us with valuable information on further relevant sources and references. The basic works in particular were well reproduced. However, less popular sources or articles on specific topics were often untraceable and in some cases obviously invented. Nevertheless, the tips were sufficiently inspiring for our own further research.
For our topic, we manually identified some features in the material (e.g. “temporal and spatial delimitation”) and asked ChatGPT for suggestions for further possible features. The list we received was sometimes absurd and very long, but we also found suitable and coherent suggestions. We were reminded that transitions are always accompanied by processes of resilience development. We were able to recognize this very well in the material. We had previously marked the relevant passages as “interesting/conspicuous”, but had not yet found a more precise description for them. For example, there were many negative reviews together with phrases such as “oh, yes, but it’ll work somehow”. ChatGPT’s buzzword “resilience” gave us the appropriate indication that these statements could be interpreted as signals of cognitive adaptation strategies. Based on this information, we were then able to identify further types of adaptation in the material.
One unexpectedly positive effect was that misunderstandings with ChatGPT forced us to resubmit our requests, but to be more specific and explain what kind of response we needed. This often led to clarifying questions such as: “No, that’s too vague, we need a term that…” or “No, the answer should relate to…” This meant we had to sharpen our own ideas and description of the topic. The requirement to repeatedly adapt questions has helped us to formulate our own position more precisely.
After we had formulated initial descriptions for various topics, we handed them over to ChatGPT with the request to identify logical gaps, unsubstantiated theses and missing points. ChatGPT has produced a long list of suggestions for improvement here. We rated many of them as superficial or unhelpful, e.g. “Try to proceed empirically”. Nevertheless, topics kept cropping up that provided good inspiration for further work. For example, we were given information on further possible theoretical references to transition research, together with corresponding literature references that also fitted the bill.
AI helps as a savings partner
Using ChatGPT or Gemini as a sparring partner is very different from the idea of AI taking over the analysis completely. A key difference in this approach is our active engagement. Instead of passively receiving the analysis results from ChatGPT, we are intensively involved in the analysis process. This means that we continuously engage with the material, specify our queries and critically evaluate the answers from the AI. This active debate has the advantage that we can strengthen our own position and improve our argumentation skills.
In our experience, this also creates a different working experience with less frustration than trying to get ChatGPT to deliver correct coding or even analysis. In this setting as a sparring partner, it was not necessary for the answers to be consistently correct or precise. We were able to identify incorrect or hallucinated answers quite quickly and check them against the material. At the same time, there were “aha” moments and the joy of good suggestions, which then enabled us to formulate or sharpen our own ideas and insights in a new and complementary way. This method has proven to be extremely productive and enriching for us, as it allows us to retain control of the analysis process while benefiting from the AI’s inspiring suggestions. It is precisely the weakness of the sometimes somewhat digressive answers that proves to be a strength when it comes to generating formulation ideas. In our opinion, ChatGPT is therefore very well suited as a sparring partner. Google’s Gemini is quite a bit more eloquent with its answers and provides a slightly better variation of perspectives. While ChatGPT, for example, only refers to “different perspectives”, Gemini, for example, provides the formulation suggestion “negotiation of interpretative sovereignty”, which was much more precise in our case and provides more potential for further points of reference in the further analysis.
This approach was a good fit for our sample project, as it is a more inductive approach. With more deductive, summarizing procedures, this advantage will not be as clear.
AI has helped with data protection:
- Establishing theoretical references and references
- Describing phenomena
- Sharpening your own position
- Formulation of summaries and descriptions
Our personal conclusion for further development:
f4 transcribes automatically and continues to support manual analysis work
Yes, there is a lot of potential and for us and many others, the joy of discovery and the fun of trying things out has been awakened. From our perspective, AI solves the task of transcription very well in many cases and can also be used seriously by people without much prior knowledge and with little reference to qualitative research.
In the qualitative analysis process, on the other hand, AI raises more questions than it is able to solve specific problems in a time-saving manner or fundamentally simplify or accelerate central work steps. Against this background, all, but especially newcomers to the field of qualitative research have a further challenge to face. The questions that arise are undoubtedly important and interesting, but we simply do not see any plausible explanation here for the use and explainability of research methods and our software, as to how AI can be used in such a way that it provides support over time, delivers well-founded and reliable results and is easy to communicate. Especially with regard to people who are new to the field of qualitative research, we consider good communication of methods and easily accessible software support to be more appropriate. Therefore, f4 has implemented automatic speech recognition and supports the manual (and not AI-based) evaluation of existing text data.